“Clouds are not spheres, mountains are not cones, coastlines are not circles, and bark is not smooth, nor does lightning travel in a straight line.” (Benoit Mandelbrot, November 20, 1924–October 14, 2010)
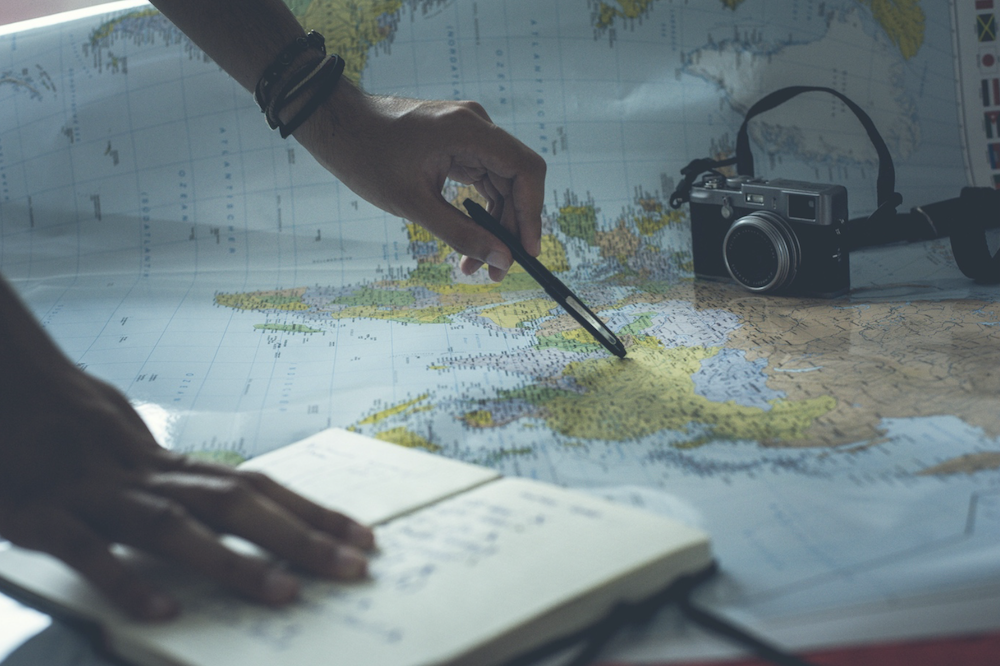
Prior to the destruction of USAID, I was working on a set of blog posts on evaluation topics and issues we face in the field. It’s not easy to think these thoughts now, with so much upheaval, uncertainty, and straight-up suffering. For me, it helps to write, and to be in touch with colleagues, so I’ll continue to share these at intervals. I hope the recent legal ruling against the destruction of USAID is enforced vigorously and soon, and that we start to unwind the betrayal, unnecessary personal tragedies, and mindless evisceration of the agency. In the meantime, let’s converse.
The map is not the territory
There is a lot of uncertainty in international cooperation projects, even before the malicious idiocy of the new administration. The only thing they’ve said that is partially – inadvertently – correct is that some projects haven’t had the results they intended. Well, no, Sherlock Musk, because this ain’t building a car. Development projects aren’t simple (see my previous post on complexity) or guaranteed.
If the tasks aid agencies try to accomplish were easy, cheap or readily replicated, they would have been done a long time ago. But they’re not. Evaluation under such circumstances must reliably show whether projects work or not (accountability) and how and why they do or do not (learning). Agencies looking for evaluators often say they require rigor in their designs and analyses, so they can be more sure of the results. But evaluating faces the same circumstances and conditions that make the projects complex and difficult. Simply adding a sheen of “rigor” (by which they often mean quantitative, especially experimental, results) doesn’t necessarily make an evaluation better: sometimes it takes up time that could be more usefully spent pursuing more qualitative answers.
Scientific rigor works well when we (evaluators, or the agency, or the implementer) can control conditions. That is very uncommon in international cooperation projects.
Moreover, we think of rigor largely in quantitative terms. In the last presidential administration, USAID brought on board a Chief Economist, whose bona fides in randomized controlled trials (RCTs) were indisputable: he was on the team that launched Innovations for Poverty Action at MIT. USAID was at the forefront of a renewed push for more RCTs and meta-analyses in development research. (I can’t speak to the current employment status of this Chief Economist, except to say that paltry few still have their jobs. Again, hopefully the latest legal ruling will start to unmake this mess.)
But RCTs make up a tiny fraction of development evaluations. This is not because evaluation commissioners lack knowledge or because RCTs are too much work. It’s because RCTs have questionable applicability in many realms of international cooperation programs. An RCT can only measure one aspect of impact, gives little to no information about the how or why, and requires some comparator – either over time or with comparison research subjects. That might mean schools or clinics with and without a project, for example.
But when the project works with a ministry to reduce corruption? Or to improve institutional efficiency? Or to change behaviors? Many times, there is no comparator. You can’t use the ministry in another country because the policies and laws governing the ministry there are too different from the treated ministry. But this challenge to comparability even happens within a given country: I’ve got an example below that talks about that.
Impact evaluation rigor in the real world
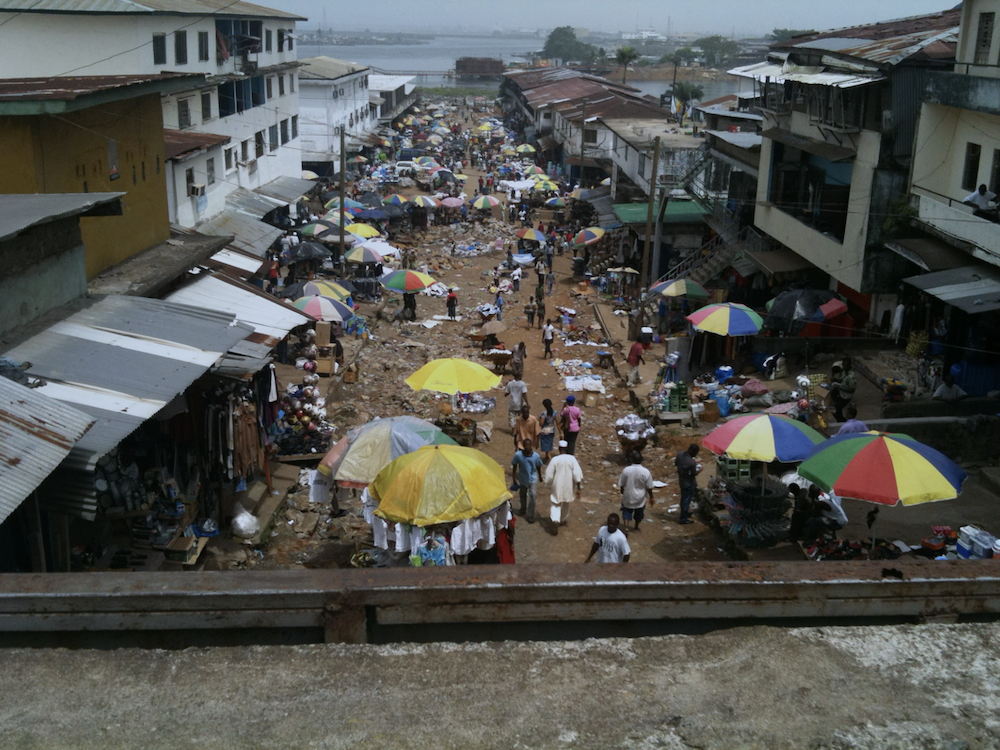
I’ve seen how impact evaluations play out in practice for the agency, and it’s disheartening. In my experience, even when the designs work well (and they often do not), the results are unimpressive for the country officers who patiently await them. They may not know how to interpret the results independently. Often, the amount of quantifiable “impact” is less than they were hoping, or even negative. This isn’t necessarily because the project didn’t have any impact. Rather, it’s because in the interim there were elections, peace negotiations started or stalled, the areas had flooding and mudslides. Impact evaluation design often can’t carve that out of the results to say what is attributable to the project, and what is attributable to external factors.
That’s true even with a control group. Trends and events can affect control or comparison groups differently, like the conflict or climate examples above. But some evaluation methods and practitioners make the promise that comparison will “control for” any differences. Researchers use what look like rigorous methods to select or sample, like creating an index of key factors and building that index for each site. The evaluators then pair units with similar index scores into treatment and control. But it’s like looking at a map and thinking it’s an accurate rendition of the territory it shows: cooperation project sites are complex and dynamic. Choosing seven or ten or twenty-five variables and combining them, however thoughtfully, looks good in the methodology section but doesn’t always match sites well.
“The textbook theory of expected utility does have one considerable virtue, which is that it’s easy to explain in textbooks.” Peter Coy, New York Times, 24 October 2022
An example of “rigor” in matching
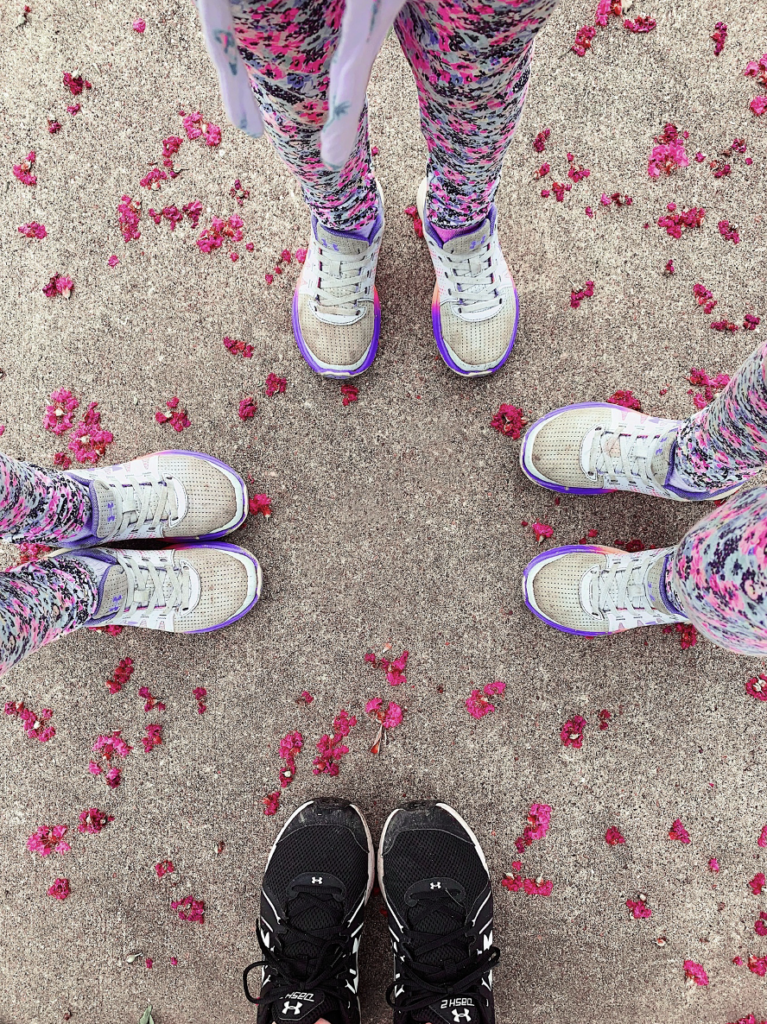
Here’s an example from my experience. In a country burdened with internal armed conflict among multiple actors, a team of university professors designed a study to measure outcomes of a program to help residents in conflict zones. After USAID paid for a considerable amount of time and data to create the index, the professors shared a list of matched villages. In each pair, one village received the program benefits, and one didn’t but had important similarities that made them a “match.”
Except that the implementing partner staff looked at the matches and said that these villages didn’t match – that, on key variables, they were very different. These differences included what kinds of income and how much income the villagers survived on, for example, or how much the armed conflict affected them, the villages’ access to markets for their produce, the role of religion in community life, or other non-trivial variables. Non-trivial, and not easily quantifiable. Comparisons between poorly matched treatment and control villages could skew the results.
But the professors said the model worked, and that the quasi-experimental design and sample size would ensure the results would be intelligible and useful. The argument is that big societal/economic/political changes would affect both treated and non-treated sites, so any difference would be attributable to the project and the project alone. The agency trusted this assessment and ran the baseline study, then signed off on the professors’ report.
Then the funders did something even weirder, but unfortunately also quite common: they hired someone else to do the midline and endline data collection. The new team arrived to find this unresolved question mark in the design, and a short timeline to collect and report on midline data – including (the agency dearly hoped) impressive impact figures! This second firm had to understand the logic, and carry out the study as previously designed. This introduced another element of uncertainty.
And so, what did they find?
No impact emerged in the data at midline, despite comprehensive theories of change, dedicated local professionals, and years of work. Whether we reviewed the data with a microscope or a telescope, there was just nothing there – no evidence that the treatment sites had any better results at all than the control sites.
I suspect that there were unobserved differences in the armed conflict in treated and non-treated villages, and/or economic differences between villages and their markets. Having different researchers at midline than baseline could also have introduced unobserved differences. But in the end, matching didn’t work. It masked heterogeneity instead of resolving it. There were too many hidden but key differences between treated and non-treated sites so that the comparison crashed.
Then again, maybe the matching was perfect and the intervention simply wasn’t any good. We’ll never know, because the study wasn’t designed to find out the how and why of successes and failures.
In another experience, I listened as an academic told the U.S. State Department that he had designed not a gold-standard RCT, but one that was platinum standard. Wow! This was in Afghanistan, and his bigger-better-brighter-and-more-rigorous design included a triple blind (not just double!) or some such nonsense. He said that made it even stronger as proof of project impact.
Is anyone out there laughing in disbelief? Does a war-strangled country seem like an appropriate place to practice unproven methods? Shortcut to the punchline: the State Department people were intrigued, and they let the fellow run his experiment. It failed spectacularly as a method, in the midst of wartime uncertainty of wartime logistics and movements of people. And the method also showed no results.
This fellow was from Yale. I’ve worked with academic researchers from Harvard and Princeton too. They know so much and are so impressive – great public speakers, so many studies behind them, often a huge research team. But it’s crucial that they have the same goal as the evaluation team – bringing back usable evidence to improve the project. If they don’t have that goal, then they’re looking at something other than the evaluation – getting field experience, prestige, publication, tenure, proving or disproving a method. Those are fine goals. But they’re not helping the evaluators reach the evaluation goals.
But Keri, how can you be against RCTs?
That’s just not true! I think they’re fascinating to work on and tremendously useful. Done well, they can make the case for cost-effectiveness and replication. Insecticide-treated bed nets are a famous example, as are cash transfer programs. Another (former) senior USAID staffer gushes that giving cash could be better than development programs and worked to test this theory. On its face, cash benchmarking sounds great – and avoids high project overhead costs.
But the majority of USAID’s work is not in providing bed nets or cash transfers, nutrition programs and the like. USAID works more and more on systems, as discussed in a previous post. Those systems require multi-faceted approaches to change, not a dosed treatment that can be measured as in a drug trial. Funders are also working to increase green energy financing, help governments renovate their infrastructure, modernize IT systems, fighting corruption, improving education quality in rural areas, and making judicial systems fairer for all. These are not environments, topics, outcomes or complexity levels that RCTs or cash benchmarking can hope to measure. When the unit of intervention is a government agency, where is the control or comparison?
I’m not against RCTs. I just think it’s a fair amount of hubris to think you can design scientific rigor, comparability, or clear cost-benefit relationships for the great bulk of cooperation projects, just because the funder wants you to.
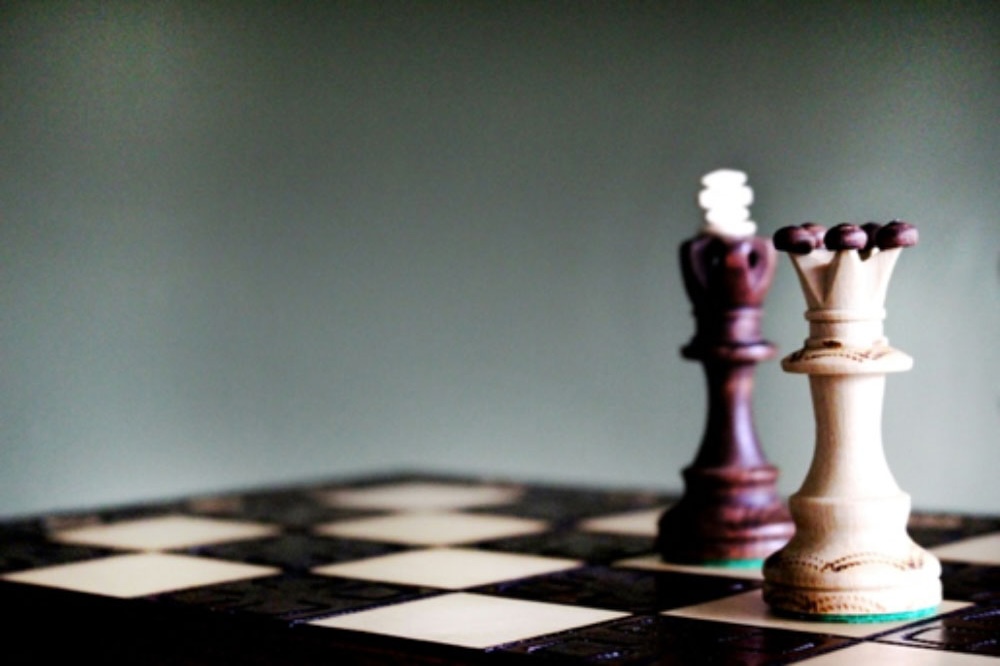
Chess has an 8 X 8 square board, two players, and six types of pieces. Even so, it has so many permutations that even the best players are hard-pressed to choose wisely from among their options. Why do we expect to reduce international cooperation projects – with un-level playing fields, draconian resource levels, hierarchical social relations, and wildly varying stakeholders and stakes – to a “difference-in-differences” calculation?
What else is there, apart from “rigor”?
“…to live with the untrammeled openendedness of such fertile not-knowing is no easy task in a world where certitudes are hoarded as the bargaining chips for status and achievement.” Maria Popova in The Marginalian, paraphrasing Wislawa Szymborska
“…a world bedeviled, as Rebecca Solnit memorably put it, by ‘a desire to make certain what is uncertain, to know what is unknowable, to turn the flight across the sky into the roast upon the plate’.” Ibid
There are no formulas that will tell us clearly, firmly, finally and flatly what works and what doesn’t. We have to wade into the uncertainty and rest comfortably with not knowing, and with failure. These are not characteristics that international aid agencies, or most regular people, particularly enjoy.
Aid agencies can do better, however. They painstakingly review multiple evaluation proposals, references and writing samples, and decide after careful deliberation to select a given evaluation team. Then, they’ve got to trust their own decision-making process.
Most evaluators that make it through these selection processes have good experience in the thicket of ambiguity. For us it is normal to face a steep and fast learning curve, tightly limited budgets, and multi-faceted, complex projects to address intractable problems, all within unpredictable conditions. We are eager to answer your evaluation questions thoroughly, which is why we respectfully recommend fewer, better, and more realistic questions.
“More rigor” in a design can sometimes translate to ignoring substance in favor of high-gloss quantitative figures. When it is impossible to have certainty, look for strength in the face of complexity. That means rich documentation, story-telling in aid recipients’ own voices, greater detail on process, and comparative analyses that reveal what works and why.
There is no strictly scientific, unassailable, precision process to capture, explain, and rate complex human endeavors. Shame on us as evaluators if that’s what we promise in our proposals. But instead of rueing the human imperfection of an evaluation team, notice the way we look for the moment when our qualitative data stops showing new things, and how we make meaning of what we find among those diverse voices. Instead of showing you all the superficial ways a project might be replicable, notice how evaluators apply critical thinking to different situations differently, highlighting what’s unique to the place and people where the project is active. Instead of looking for ways to showcase when implementers exceed targets for numbers of people trained, celebrate those projects that are willing to fail and learn from it, undaunted.